
What is AI?
Artificial Intelligence (AI) is a field of computer science focused on creating machines or software that can perform tasks that typically require human intelligence. These tasks include learning, reasoning, problem-solving, understanding language, and recognizing patterns.
Here's a simple breakdown of what AI is all about:
- Learning: AI systems can learn from data. Just like humans learn from experience, AI algorithms improve over time by being exposed to more data. This process is often referred to as machine learning, where the "machine" gets better at a task by being exposed to more examples.
- Reasoning: AI can make decisions based on the information it has learned. This involves weighing different pieces of information, considering past experiences, and then making decisions based on this analysis, much like how humans make decisions.
- Problem-solving: AI can be used to solve complex problems that are difficult for humans to solve quickly. This includes everything from figuring out the best route to take to avoid traffic, to diagnosing diseases based on symptoms and medical history.
- Understanding Language: AI can be trained to understand human languages, allowing it to communicate with people, understand written texts, and even generate human-like text. This aspect of AI is often referred to as natural language processing (NLP).
- Pattern Recognition: AI is good at recognizing patterns in large sets of data. This can be anything from identifying faces in photos to detecting fraudulent activity in financial transactions.
Recent developments in AI over the past year have showcased significant trends and shifts in the field:
- Generative AI in Business: Generative AI tools have seen widespread application in marketing, sales, product development, and service operations. Despite high expectations for its impact, many companies are not fully prepared for the risks, with a minority addressing issues like inaccuracy or cybersecurity. However, AI high performers, or companies deriving significant value from AI, are using generative AI more broadly and effectively, focusing less on cost reduction and more on creating new business opportunities.
- Customized Enterprise Generative AI Models: Businesses are increasingly adopting smaller, customized AI models tailored to specific needs rather than using massive, general-purpose models. This trend is driven by the need for AI systems that cater to niche requirements and is significant for sectors with specialized terminology like healthcare, finance, and legal.
- Humanoid Robotics: The introduction and integration of Generative AI, particularly Large Language Models (LLMs) like GPT-3.5, into the robotics industry have opened new possibilities for human-robot interaction. Companies like Agility Robotics have begun using LLMs to control robots such as Digit, enabling these machines to understand and follow natural language commands. This development has led to rapid prototyping and deployment of AI-powered robots, with plans for commercial testing and production scaling up, such as Agility Robotics' announcement of Amazon testing their humanoid robot and their plans to produce up to 10,000 robots annually.

How Is AI Affecting Traditional Risks?
One thing is for certain - AI has made a dent in the world and is here to stay. How does insurance adapt to the new world? Today, we are exploring how AI affects traditional risks and what both companies and insurers need to be on the lookout for.
Generative AI and SaaS Applications
Generative AI, especially in Software as a Service (SaaS) applications, has revolutionized how businesses operate, offering unprecedented efficiency and customization. However, this advancement brings with it a host of risks. The primary concern lies in data security and privacy. As generative AI models require massive datasets to learn and generate responses, they often ingest sensitive information, leading to potential data breaches. For instance, if a generative AI chatbot in a customer service application learns from confidential customer interactions, there's a risk of inadvertently generating responses that reveal personal information to unauthorized users.
Moreover, there's the issue of reliability. Generative AI can produce results that seem plausible but are factually incorrect, a phenomenon known as 'hallucination'. This can lead to misinformation if, for example, a financial advice application provides incorrect investment suggestions.
Foundation Models
Foundation Models, like OpenAI's GPT series, underpin many AI systems, offering a broad understanding of language and concepts. While they're incredibly versatile, they're not without faults. One significant risk is the propagation of bias. These models are trained on vast swaths of internet data, reflecting and amplifying existing societal biases. This can result in unfair or prejudiced outcomes, particularly in sensitive applications like hiring tools or loan approval systems.
Another risk is overreliance. Businesses may depend heavily on these models without fully understanding their limitations, leading to flawed decision-making processes. For instance, relying on a foundation model for critical health diagnoses without proper oversight can lead to misdiagnoses or overlooked symptoms.
Robotics
The integration of AI into robotics has led to more autonomous and intelligent machines capable of performing complex tasks. However, this integration introduces risks, particularly in safety and job displacement. In manufacturing, for example, a robot malfunctioning due to an AI error can cause accidents, endangering human workers.
Additionally, as robots become more capable, they can displace human jobs, leading to economic and social challenges. While automation can increase efficiency, it also raises questions about the future of work and the potential for widespread joblessness in certain sectors.
Autonomous Vehicles
Autonomous vehicles (AVs) epitomize the intersection of AI and real-world applications. They promise to revolutionize transportation but also introduce several risks. Safety is a paramount concern; AI systems can fail to interpret road conditions correctly, leading to accidents. Incidents involving self-driving cars have highlighted the limitations of current AI technologies in navigating complex real-world scenarios.
Furthermore, there's the risk of security vulnerabilities. AVs rely on AI systems that are connected to the internet, making them potential targets for cyberattacks. A hacked vehicle could be commandeered, leading to disastrous outcomes.

What Impact Does AI Have on Traditional Insurance Coverage?
As AI becomes more integrated into daily life, insurance companies and policyholders are facing new challenges. Specifically, the biggest question is, what happens when AI does something wrong? In other words, who is liable for AI's mistakes - the user or the developer?
What Is Not Covered?
AI introduces complexities in determining liability and coverage due to its autonomous decision-making capabilities. Traditional insurance policies were not designed with AI's unique risks in mind, leading to gaps in coverage. For instance:
- Data Breaches and Cybersecurity Threats: As companies leverage AI for data processing and storage, they become targets for cyberattacks, which may not be covered under standard insurance policies.
- AI Bias and Ethical Issues: If an AI system's decision, influenced by inherent biases, leads to discriminatory practices or wrongful denials (such as in loan applications or job screenings), businesses could face lawsuits not necessarily covered by traditional liability insurance.
- Autonomous System Failures: In sectors like automotive or healthcare, where AI systems can operate independently (e.g., autonomous vehicles or diagnostic tools), determining liability for malfunctions or accidents can be complex. Traditional policies may not clearly define coverage for these scenarios.
- Intellectual Property Risks: AI can generate content or designs that infringe on existing copyrights or patents. Traditional insurance might not cover the legal costs associated with IP infringement claims.
Examples
Let's delve into some examples to illustrate these points:
- Cybersecurity Threats: Consider a scenario where an AI-powered system is hacked, leading to significant data loss. A traditional business liability insurance policy may not cover the damages if the policy excludes cyber risks. Companies now often need to obtain specific cyber insurance policies to mitigate this risk.
- AI Bias: An AI recruitment tool might inadvertently favor candidates of a certain demographic, leading to discrimination lawsuits. Traditional employment practices liability insurance may not cover claims arising from AI-driven decisions unless explicitly stated.
- Autonomous System Failures: If an autonomous vehicle causes an accident, the question arises: is the manufacturer, software developer, or vehicle owner liable? Traditional auto insurance policies may not clearly address these new layers of complexity.
- Intellectual Property Risks: If a company uses a generative AI tool to create marketing materials that inadvertently copy another brand's content, they could face copyright infringement lawsuits. Standard business insurance policies typically do not cover such intellectual property disputes.
As AI continues to make rapid progress, legal precedents will arise and accumulate, creating a legal base for handling liability accidents. In the meantime, it is crucial for AI companies, as well as companies using AI, to protect themselves from product- and service-related claims and lawsuits, especially in today's litigious environment.
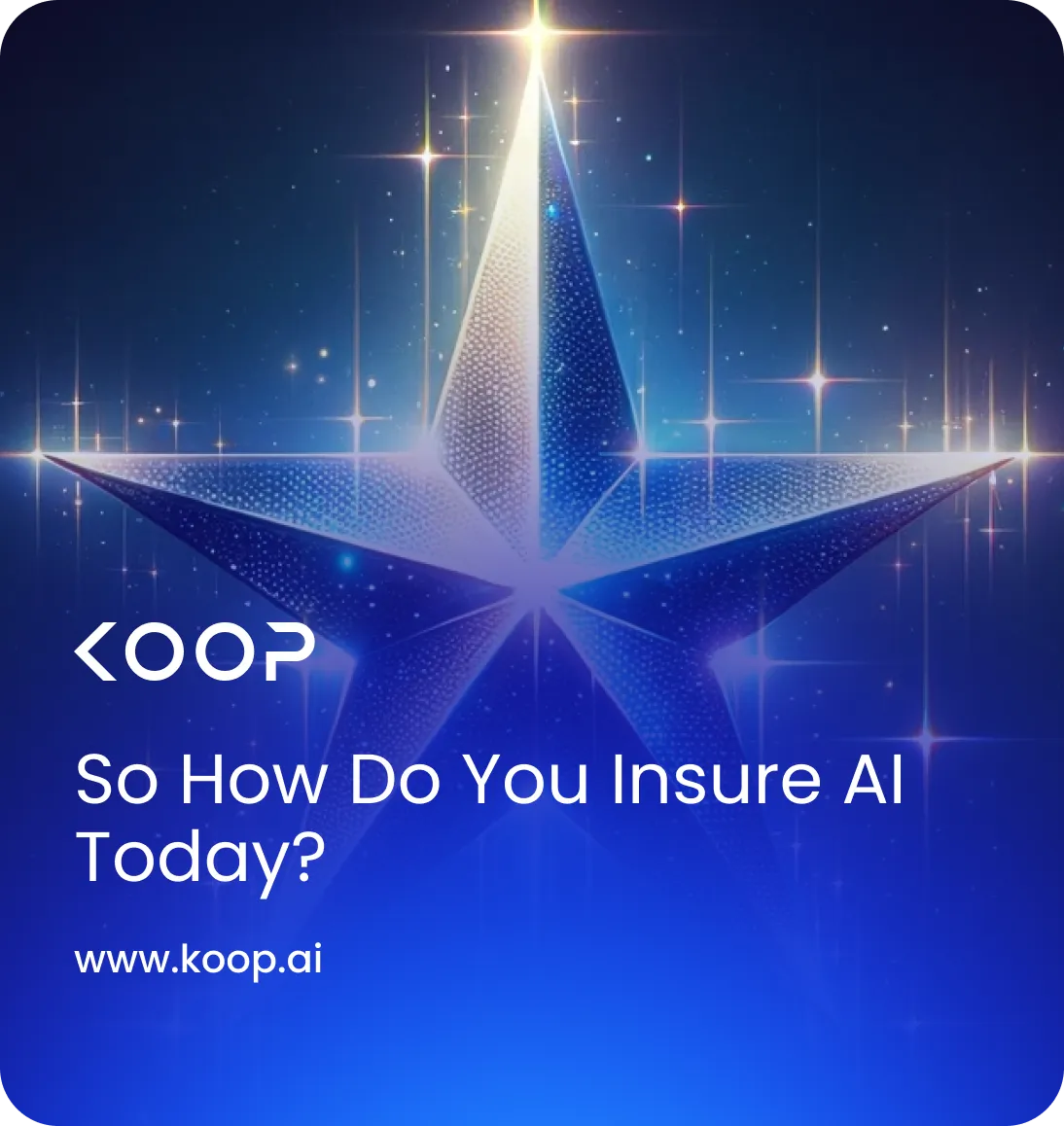
So How Do You Insure AI Today?
Even though there are coverage and legal challenges with insuring AI, it is not impossible. Here are practical tips for companies developing AI-based products and services:
- First, create a risk management program. It will help you not just procure coverage but also reduce your overall company risk. There are options like Vanta if you want to install a formal security framework such as SOC 1, SOC 2, or ISO 27001. Alternatively, you can use ERM Automation tools such as Koop, which is very time and cost-efficient control implementation and reporting.
- Second, find a specialized insurance brokerage with expertise in Product Liability, Technology Errors and Omissions, and Cyber Liability. It is recommended to purchase a package because it could be unclear which coverage would pick up an accident caused by AI. For example, if there was a data component, Cyber would typically be required. E&O would pick business interruption, while a more widespread accident with the hardware involved could trigger product liability. Always consult with your insurance advisor on the most optimal package.
- Third, ensure that the cost of coverage is adequate. Insurance premiums can easily get out of hand, especially if you deal with insurers who are not familiar with the Tech sector and AI specifically. Be sure to partner with someone who not only gets you covered but does not bug you with sudden cancelations of notice or burdensome audits.
- Finally, keep improving your technology! Whether you use your own frameworks and methodologies for AI safety or more generalist tools like Koop's ERM Automation, be sure to use that for improving coverage. Keep in mind that insurance doesn't bite until you have to file a claim, and it is always a good idea to do everything you can to prevent a claim from happening in the first place!

Resources on AI Safety
If you're looking to delve into AI safety, here are some resources that can provide you with a wealth of information:
- OpenAI's Approach to AI Safety: OpenAI offers insights into their approach to ensuring AI systems are built, deployed, and used safely. They emphasize rigorous testing, feedback from external experts, and continuous real-world learning to improve AI safety measures. This resource is particularly valuable for understanding practical steps in AI safety implementation. You can explore more about their safety measures and philosophies here.
- Center for AI Safety (CAIS): Based in San Francisco, CAIS is a research and field-building nonprofit focused on reducing societal-scale risks associated with AI. They conduct impactful research, provide resources for AI safety research, and engage in advocacy for safe AI practices. Their work is dedicated to tackling the many unsolved problems in AI safety and ensuring the technology's benefits do not come at the expense of public safety. Learn more about their mission and projects here.
- AI Safety Fundamentals Course: This is an educational resource offering a comprehensive introduction to the fundamentals of AI safety. The course covers topics like anomaly detection, alignment, and risk engineering, making it a great starting point for those new to the field. It's designed to reduce barriers to entry into AI safety and provide learners with the knowledge needed to contribute to safer AI development. You can access the course here.